Client Background
Our Client Pioneers in Precision Oncology and Genomics
Our client is a leader in precision oncology, combining advanced genomics with cutting-edge digital health technologies to redefine cancer care. To address complex cancer detection, our client has pioneered comprehensive genomic panels that decode the unique genetic blueprint of a patient’s cancer. These insights empower oncologists to craft highly personalized treatment plans, targeting specific mutations with unparalleled precision. By pushing the boundaries of science and technology, our client is shaping the future of oncology, delivering hope and transformative outcomes to patients worldwide.
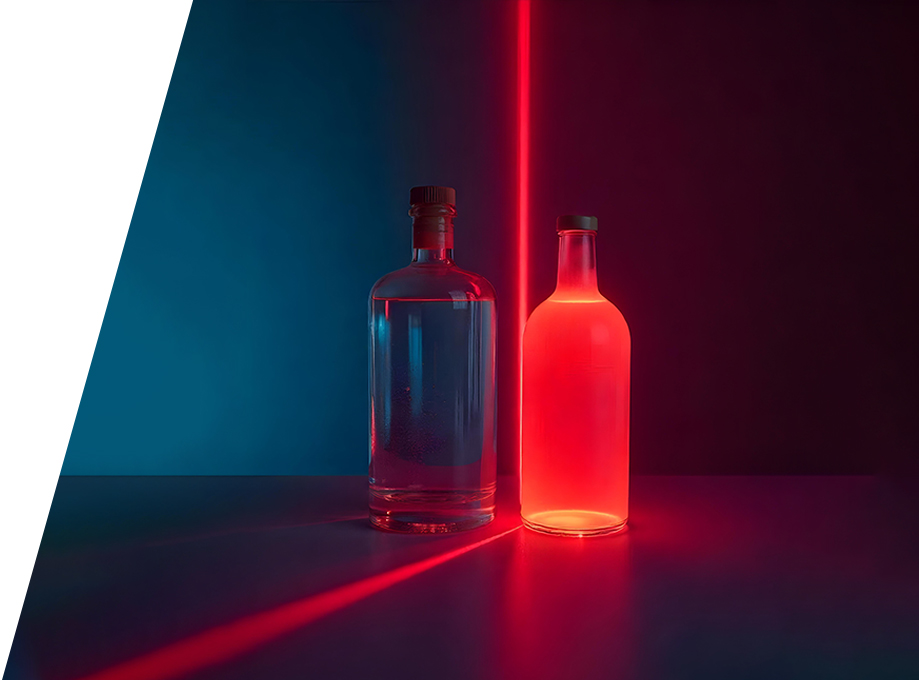
Challenge
Difficulties in Predicting Novel Variants and Identifying Cancer Genes
The client wanted to harness machine learning to address a key challenge in precision oncology for distinguishing pathogenic from non-pathogenic genetic variants. By leveraging advanced predictive models, they aimed to enhance diagnostic accuracy and deepen understanding of cancer’s genetic drivers. This effort paves the way for targeted therapies, transforming the interpretation and application of cancer-associated genetic data in clinical care.
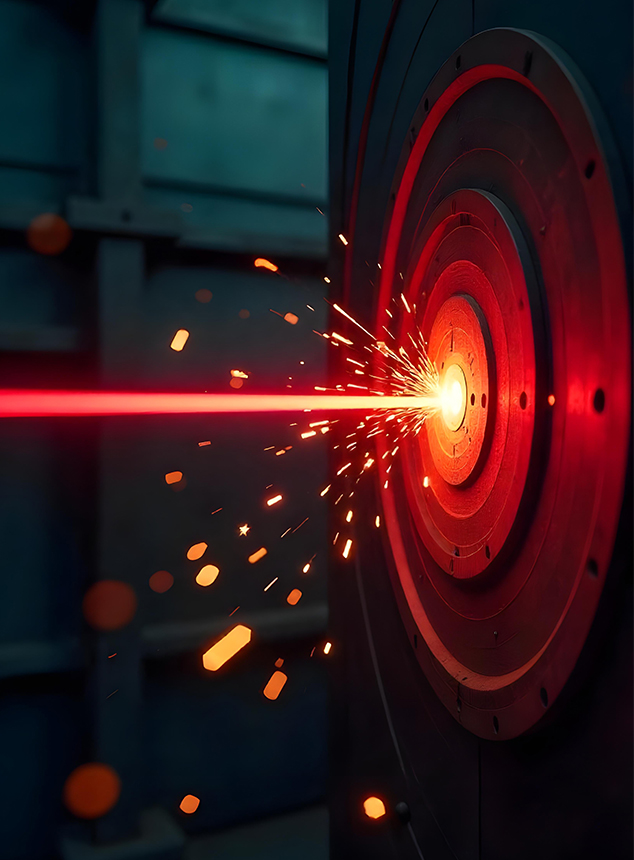
Healthark’s role
Developed ML Model for Pathogenic Variant Prediction and Driver Gene Identification
Healthark analyzed genetic variants from a lung cancer dataset and developed a machine learning model to predict pathogenic and non-pathogenic variants. This provided insights into potential cancer-causing driver genes and their associated variants.
- Data Cleaning The dataset was carefully filtered to retain only relevant data for analysis. Entries with functions outside of Synonymous and Non-Synonymous were excluded to ensure a focus on meaningful genetic changes. The amino acid change information was extracted into a separate column, and null values were removed to ensure data consistency and reliability, setting a solid foundation for subsequent analysis.
- Feature Engineering and Clustering Key features were carefully selected to identify the most impactful columns, with a target variable chosen to categorize samples into classes like Pathogenic and Benign. This enabled a focus on clinically significant outcomes. Categorical features were transformed into numerical values through one-hot encoding and key mapping, ensuring feature importance was preserved. Min-Max normalization was applied to standardize the data, promoting comparability across features. To handle chromosome-specific variations effectively, the dataset was segmented into 24 subsets by chromosome numbers.
- Modelling and Variants Prediction To predict sample clusters with an ensemble of high accuracy, machine learning models like Random Forest, XGBoost, and Extra Trees classifiers were deployed. These models were chosen for their ability to handle complex genetic datasets and deliver reliable results. Ensemble modelling was employed to combine their strengths, reducing model bias and variance while enhancing prediction accuracy and robustness.
- Sample Wise Clustering Each chromosome dataset was clustered separately to uncover chromosome-specific patterns and insights. Clustering techniques were applied to group similar samples based on their genetic features, enabling a deeper understanding of the dataset. This approach facilitated targeted analyses and highlighted meaningful genetic variations across chromosomes.

Empowering Tomorrow's Healthcare
This case study exemplifies how collaboration between CROs and specialized service providers like Healthark can empower life science companies to leverage the power of real-world data. By harnessing this powerful resource, Healthark Insights empowers life science companies to make data-driven decisions and personalize care for patients.
Want to learn more about Healthark’s expertise in Real-World Evidence? Explore our website or contact us today!