Introduction
In real-world evidence (RWE) studies, patient behavior rarely follows the structured protocols seen in clinical trials. One major challenge is treatment crossover, where patients deviate from their initial treatment path by switching therapies, discontinuing treatment, or combining interventions. While reflective of real-world clinical scenarios, these crossovers can complicate data analysis, introducing bias and diluting treatment effect estimates.
Effectively addressing crossovers in RWE studies is crucial to ensuring robust, actionable insights that guide healthcare decisions. This blog explores the causes and challenges of treatment crossover, alongside innovative solutions to maintain analytical integrity.
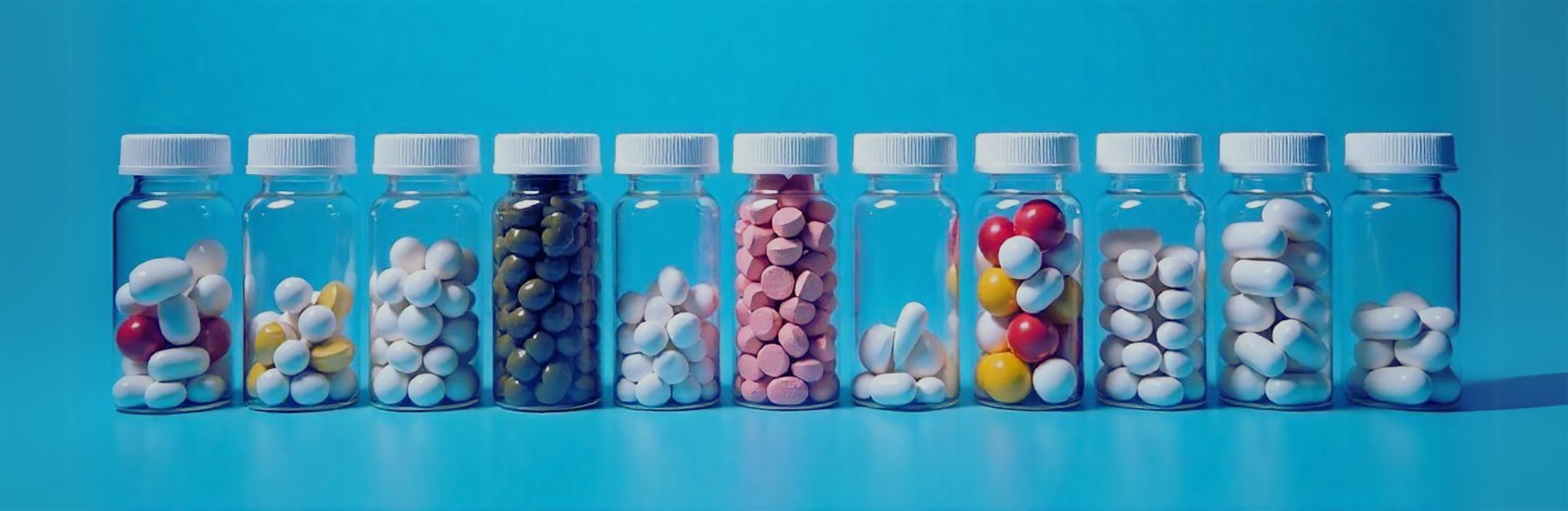
Understanding Crossover in RWE Studies
Crossover refers to patients switching from their original treatment to another, altering their therapeutic journey. Unlike clinical trials, where changes are closely monitored, crossovers in RWE often occur unplanned and can be influenced by various factors, including:
- Clinical necessity: Disease progression or adverse effects that require changes in treatment.
- Physician decisions: Updates to treatment guidelines or adjustments based on patient monitoring and outcomes.
- Patient-driven changes: Personal preferences, affordability, access to medications, or concerns about side effects.
Example: A patient with type 2 diabetes may transition from oral medications to insulin due to declining glycemic control, introducing variability into an RWE study examining long-term outcomes of oral therapy. This variability reflects real-world practice but poses challenges for analysis.
Challenges of Crossover in RWE Studies
1. Biased Treatment Effect Estimates
When patients switch treatments, it becomes harder to determine whether the original or new treatment contributed to observed outcomes. This can lead to:
- Over or underestimation of treatment effects.
- Ambiguity in linking specific outcomes to a single therapy.
2. Confounding Variables
Patients who cross over often differ systematically from those who don’t. For example:
- Patients with more severe disease are more likely to switch therapies, creating a bias in the analysis.
- Differences in adherence or socioeconomic factors may also confound results.
3. Data Fragmentation
Frequent treatment changes create fragmented pathways, complicating long-term analyses. This fragmentation reduces the clarity of insights into treatment effectiveness and makes it difficult to draw conclusions.

Strategies to Address Crossover in RWD Analysis
1. Advanced Statistical Methods
Statistical methods can adjust for biases introduced by crossover:
- Marginal Structural Models (MSMs):
- MSMs adjust for time-varying confounders (factors that change over time and affect both treatment and outcomes). By reweighting patient data, they simulate a scenario where patients didn’t switch treatments, preserving the integrity of comparisons.
- Example: MSMs have been used in diabetes studies to correct for differences in disease progression among patients switching from oral drugs to insulin.
- Inverse Probability Weighting (IPW):
- IPW assigns weights to patients based on how likely they were to stay on their original treatment or switch. This technique ensures that the comparison groups are balanced, reducing bias.
- Example: In a cardiovascular study, patients with worse baseline health were more likely to switch medications. IPW adjusted the analysis by giving these patients lower weights to prevent them from disproportionately influencing the results.
- Instrumental Variable Analysis (IVA):
- IVA relies on external factors, like geographic prescribing trends or hospital policies, as tools to estimate treatment effects in the presence of crossover. These variables must influence treatment choice but not the outcomes directly.
- Example: In a regional oncology study, geographic differences in drug availability were used as an instrument. Patients in areas with higher access to a specific therapy were more likely to switch, and IVA used this variation to estimate the treatment’s true effect while accounting for crossover.
2. Dynamic Patient Cohort Definition
Defining patient groups based on real-world treatment paths (figure 1) ensures meaningful analysis:
- Pathway Segmentation:
- Organizing patients into pathways (e.g., “only Therapy A” or “Therapy A followed by Therapy B”) allows researchers to compare distinct treatment sequences.
- Subgroup Analyses:
- Researchers examine specific subgroups, such as patients who stayed on their initial treatment versus those who switched, to understand how outcomes differ.
Figure 1: Dynamic Patient Treatment Pathways
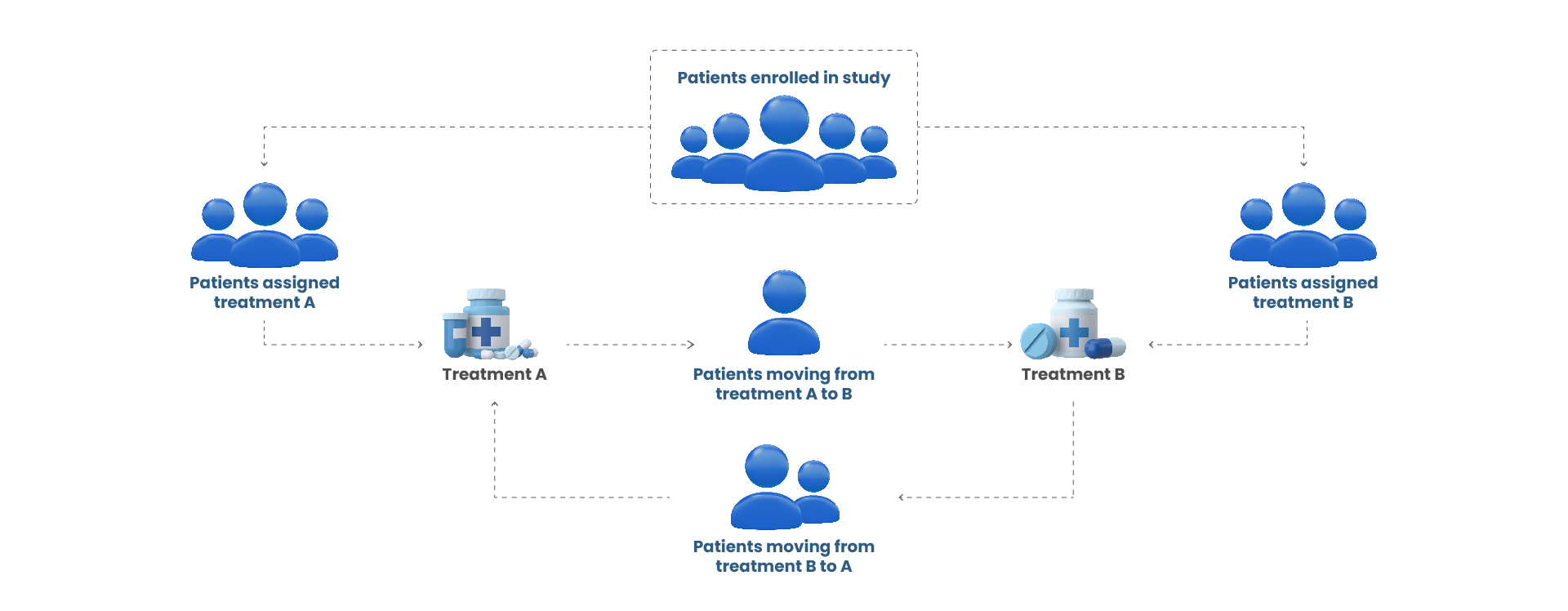
3. Sensitivity Analysis
Sensitivity analyses test how robust the study results are under different assumptions:
- Excluding crossover patients ensures that the findings focus only on patients who adhered to their initial treatment.
- Treating crossover as a covariate adjusts for the impact of switching within statistical models, improving accuracy.
- Simulating no-crossover outcomes uses predictive modeling to estimate what the results would have been if no crossovers occurred.
4. Leveraging Emerging Technologies
Advanced technologies offer innovative solutions to manage crossover challenges:
- Machine Learning (ML):
- ML predicts which patients are most likely to switch treatments, helping researchers plan analyses or focus on certain groups.
- Example: In oncology, ML algorithms identified patients who were likely to switch therapies after experiencing early side effects, aiding targeted adjustments.
- Synthetic Data Augmentation:
- Synthetic data creates simulated populations, especially useful in rare diseases where crossovers might deplete sample sizes.
- Real-Time EHR Monitoring:
- Regular tracking of EHR data documents crossover events as they happen, providing more accurate insights into how and why patients change treatments.
Conclusion
Handling treatment crossover is one of the most significant challenges in real-world data analysis, but it also mirrors the complexities of clinical practice. By employing advanced statistical techniques, clearly defining treatment pathways, and leveraging emerging technologies, researchers can ensure accurate, unbiased insights while embracing the dynamic nature of real-world healthcare.
These strategies not only strengthen the validity of RWE studies but also enhance their value in informing clinical and policy decisions. Successfully addressing treatment crossover will lead to more actionable insights, ultimately benefiting patients, providers, and policymakers alike.