In an era where technological advancements are revolutionizing every facet of our lives, the healthcare industry stands at the forefront of innovation. One of the most promising developments in recent years is the integration of Artificial Intelligence (AI) into clinical trials. These technologies are reducing development time and costs while providing data-driven insights that facilitate faster breakthroughs and more informed decisions.
According to Roots Analysis, the global AI in clinical trials market size is estimated to grow from $1.42 billion in 2023 to $8.5 billion by 2035, representing a compound annual growth rate of 16% during the forecast period 2023-2035.
Figure 1: Global AI in Clinical Trials Market Size (In Billions) (2023 to 2035)
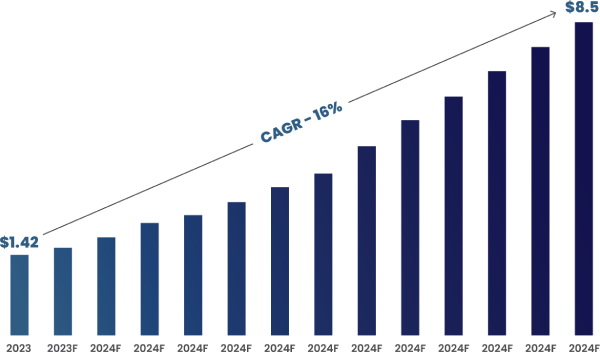
The Traditional Approach to Clinical Development
Clinical development is a complex and resource-intensive process that involves multiple phases, from preclinical testing to post-marketing studies. Traditionally, this process has been characterized by meticulous planning significant investments, and extensive time requirements, often spanning over a decade and costing billions of dollars. However, despite these efforts, the success rate of bringing new treatments to market remains relatively low, with many potential therapies failing to demonstrate efficacy or safety during clinical trials.
Figure 2: Current Landscape of the Clinical Development Cycle

Developing a new drug and bringing it to market typically takes up to 15 years, with an average total R&D expenditure ranging from 1.5 to 2 billion USD. Approximately half of this investment is allocated to clinical trials, with Phase III trials being the most complex and costly. The probability of success for compounds advancing through the clinical trial stages varies by phase, resulting in 1 out of 10 compounds entering clinical trials ultimately receiving FDA approval. This high clinical trial failure rate significantly contributes to the inefficiency of the drug development cycle.
Two major factors contributing to these high failure rates are poor patient cohort selection and ineffective recruiting mechanisms, along with the challenges in monitoring patients adequately during trials. Recent statistics reveal that 25% of studies fail due to inadequate patient enrollment, with 50% of sites enrolling either one or no patients in studies.
To address these issues, companies and qualified medical institutions are increasingly adopting patient-centric approaches to recruit and engage with trial participants. Digital tools, such as mobile apps and social media, and collaborative efforts are being utilized to establish patient-centric trial patterns. These methods aim to enhance access to clinical trials, reduce the burden on patients, increase participant diversity, and expedite the approval of breakthrough therapies.
Emerging Role of AI and Real-World Data (RWD) in Transforming Clinical Development
The adoption of Artificial Intelligence (AI) and Real-World Data (RWD) in clinical development is expanding significantly, driven by the increasing availability and diversity of data sources. Beyond traditional electronic health records and claims data, emerging sources such as wearable devices, mobile health applications, and social media platforms are enriching the dataset available for analysis. These sources offer real-time insights into patient behaviors, treatment adherence, and lifestyle factors, providing a holistic view of patient health outside clinical settings.
One of the key challenges in clinical development has been the extraction of information from unstructured data, such as physician notes, medical imaging reports, and scientific literature. While this data is rich in valuable information, it is often difficult to analyze and integrate into research workflows. However, advancements in AI, particularly in natural language processing (NLP) and image recognition, are making significant strides in converting unstructured data into structured, actionable insights. For instance, NLP models can now accurately parse through vast amounts of text, extracting key clinical information and converting it into structured data formats that can be readily utilized in research and analysis.
The integration of structured and unstructured data offers a comprehensive understanding of patient experiences, treatment efficacy, and adverse events, thereby enhancing decision-making throughout the drug development lifecycle.

Applications of AI in Clinical Trials
- Patient Recruitment and Enrollment
AI-powered solutions are revolutionizing this process by leveraging data-driven approaches to swiftly identify and match eligible patients with clinical trials. For instance, predictive modeling algorithms analyze a patient’s symptoms, medical history, geographical location, and provider capacity to identify the best match for a particular study. By mining vast datasets from EHRs and provider databases, AI platforms can rapidly analyze both structured data, such as ICD codes, and unstructured data, including prescriptions and diagnostic reports. This accelerates the patient matching process, reducing waiting times from weeks to days.
- Optimizing Clinical Trial Design and Protocol Development
Machine learning algorithms are increasingly being employed to optimize clinical trial design and protocol development, thereby enhancing the efficiency and cost-effectiveness of the drug development process. By analyzing large-scale EHRs, thesealgorithms can assess the capacity of potential trial sites and accurately estimate the number of eligible patients available for enrollment. This proactive approach helps to prevent situations where sites falsely claim to have sufficient patient populations, reducing wasted resources and meeting tight trial deadlines.
- Site Identification
Identifying suitable sites for clinical trials can be challenging due to limited patient populations and logistical constraints. AI technologies offer a solution by analyzing vast amounts of data from EHRs, claims databases, and other sources to identify potential trial sites with the requisite patient populations and infrastructure. Machine learning algorithms can assess various factors such as patient demographics, disease prevalence, physician expertise, and geographic location to identify sites that are best suited for specific clinical trials. This streamlines the site selection process, optimizes resource allocation, reduces trial costs, and accelerates clinical development timelines.
- Remote Patient Monitoring Clinical Data Capturing
Remote patient monitoring (RPM) has emerged as a powerful tool for collecting real-time data on patient health and behavior outside of traditional clinical settings. RPM solutions leverage biometric sensors and wearable devices to monitor patient vital signs, medication adherence, and disease symptoms. This data is transmitted to healthcare providers in real-time, allowing for early detection of adverse events and timely interventions. AI algorithms can analyze this streaming data to detect patterns, identify trends, and generate predictive insights that inform clinical decision-making. This approach captures rich, real-world clinical data, enhancing the understanding of disease progression, treatment efficacy, and patient outcomes, ultimately leading to more informed drug development decisions.
- Personalized Medicine and Biomarker Discovery
AI and data analytics play a crucial role in driving personalized medicine initiatives by enabling the discovery and validation of novel biomarkers. By analyzing large-scale omics data, including genomics, proteomics, and metabolomics, AI algorithms can identify subtle molecular signatures associated with disease phenotypes and treatment responses. These insights facilitate the development of biomarker-based diagnostic tests that enable more precise patient stratification and personalized treatment recommendations.
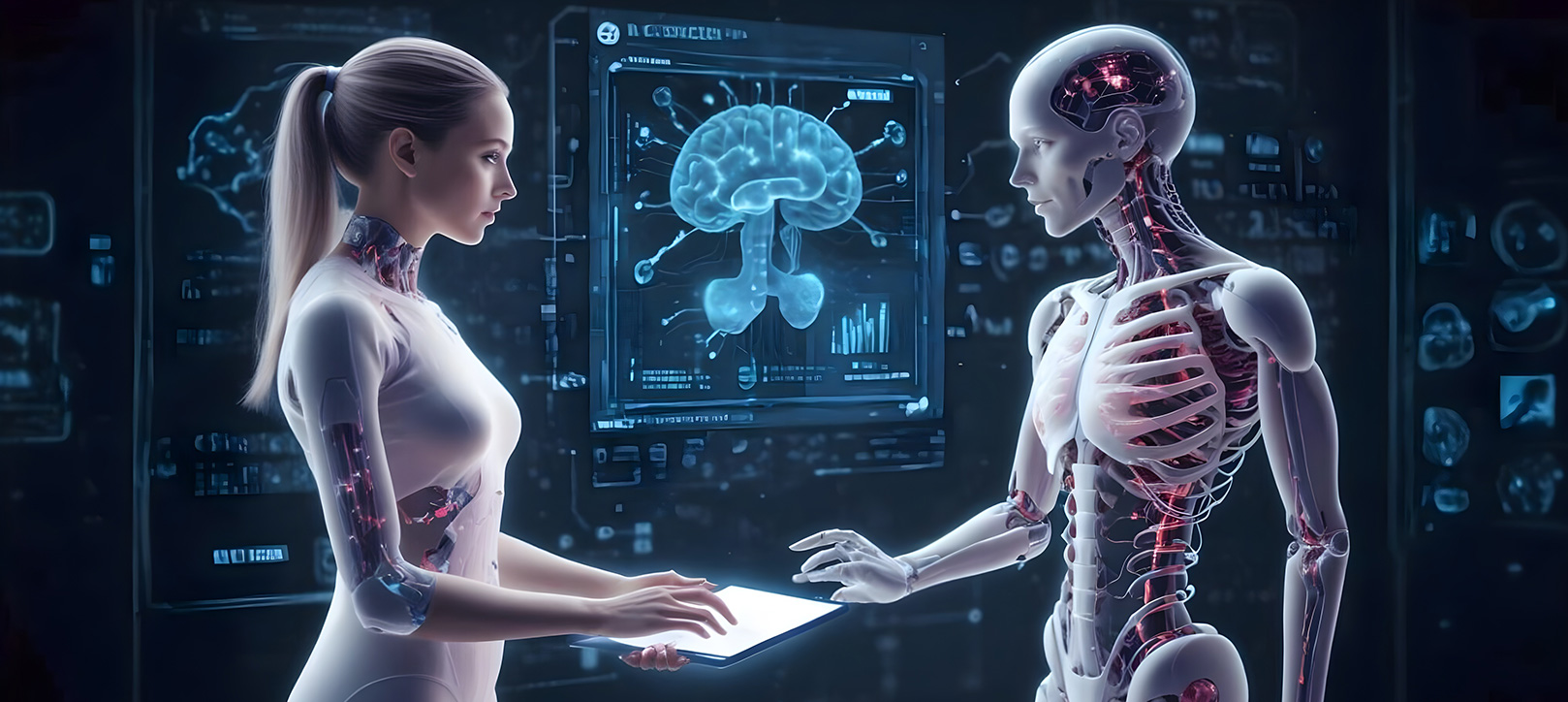
Conclusion
The adoption of Artificial Intelligence in clinical trials is revolutionizing healthcare. It enhances patient recruitment efficiency, optimizes trial design, and enables real-time data collection through remote patient monitoring. AI-driven approaches promise accelerated drug development timelines, cost reduction, and personalized medicine initiatives. Ultimately, this integration offers the potential for faster breakthroughs, more informed decisions, and improved patient outcomes.